Herbsttagung 2019
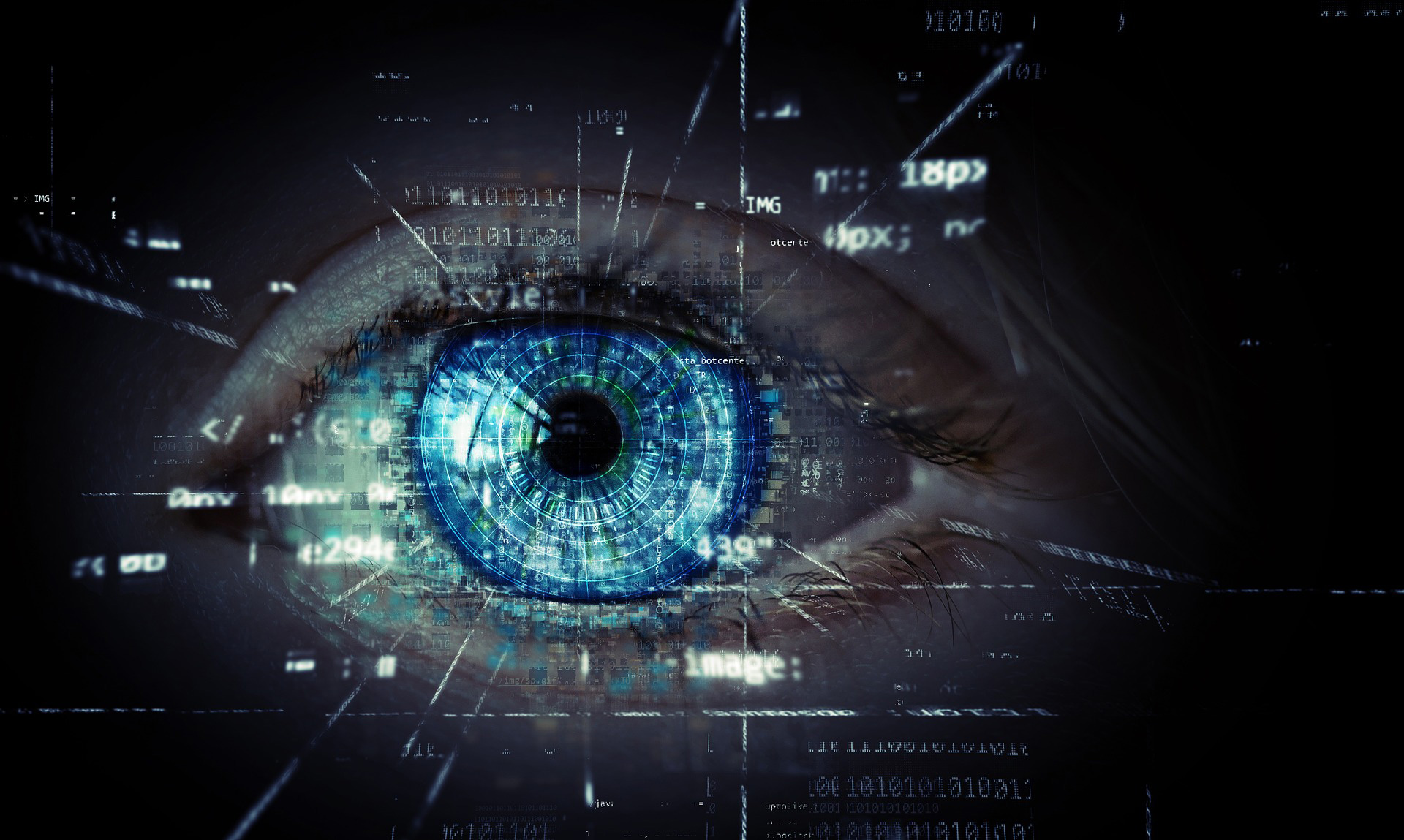
Explainable and trustworthy AIRecap of our autumn conference
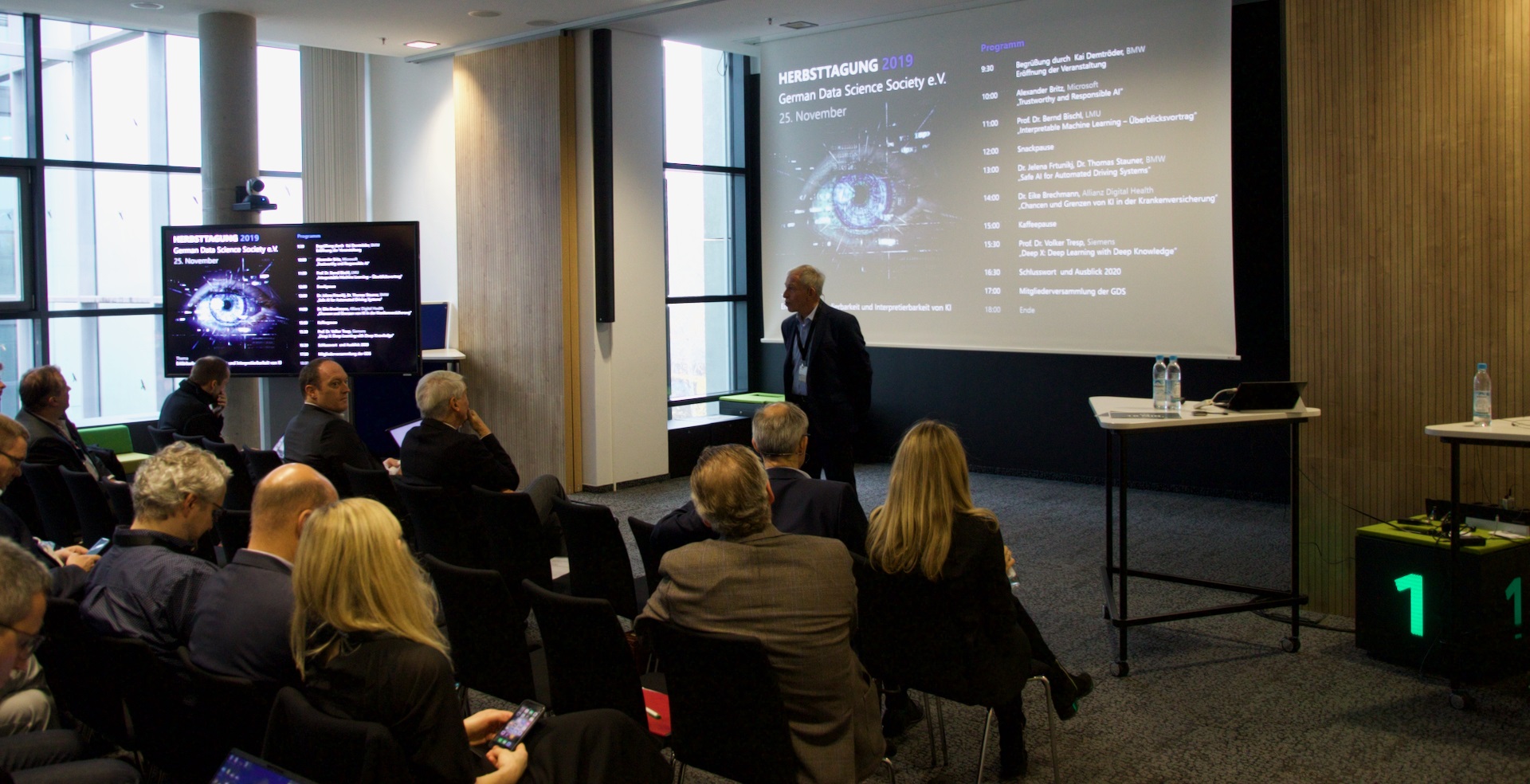
Our 2019 autumn conference focused on „Explainability, Interpretability and Regulation of Artificial Intelligence”.
Here is a summary of the most important points, that is essentially based on a blog of Lena Prummer, Communications Manager Data Analytics & AI at Allianz.
Welcome Speech by Kai Demtröder (BMW)
Explainability, interpretability, regulation, ethics, and security are core topics that drive data science research and practice. They are crucial for building robust and useful solutions, and are key to a healthy discussion with the general public.
One of the key challenges for BMW is the industrialization of AI: How do you scale an AI solution from a couple of users to several thousand without compromising quality, effectiveness and confidence on the part of users?
Trustworthy and Responsible AI by Alexander Britz (Microsoft)
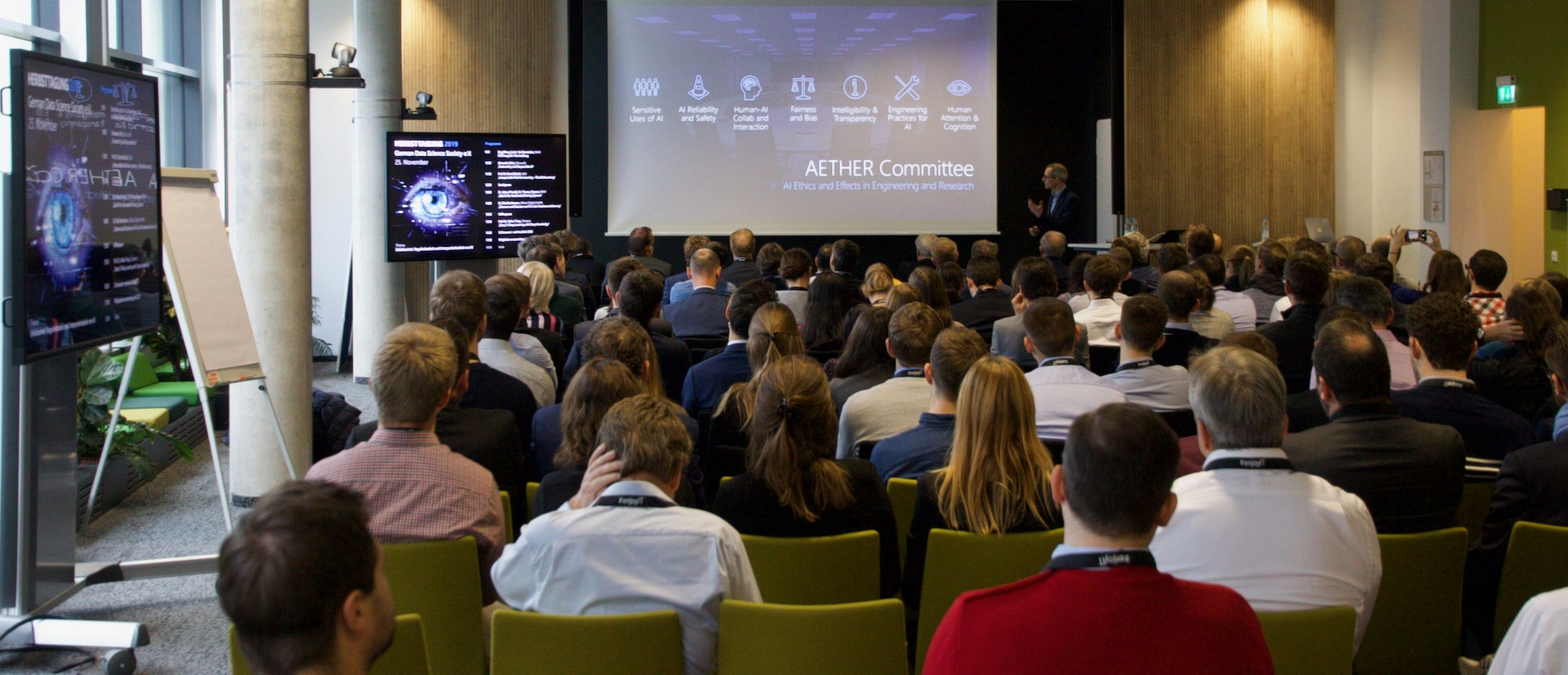
Alexander Britz opened his speech by highlighting the importance of ethical development of AI to avoid unintended or questionable outcomes. The use and development of facial recognition is one example, the risk of implementing unconscious bias another. When a simple search for the term “CEO” only produces images of white men, a limited underlying data set could be the reason. He also refers to Microsoft’s own example of a chatbot that turned racist and misogynistic in a matter of hours, when it “learned” from feedback the users provided. The chatbot was taken down barely 24 hours after it launched in 2016. Since then, a lot has happened in the field of responsible AI, amongst this, the company developed principles for responsible AI.
Microsoft’s six principles for responsible AI: Fairness, Reliability, Privacy & Security, Inclusiveness, Transparency, Accountability
One example of how Microsoft acts on these principles: The company has installed the AI and Ethics in Engineering and Research committee (AETHER) bringing together senior leaders from across the company to focus on proactive formulation of internal policies and how to respond to specific issues in a responsible way. Employees who feel uncertain about potential businesses can address the committee and raise visibility.
Interpretable Machine Learning by Bernd Bischl (LMU University of Munich)
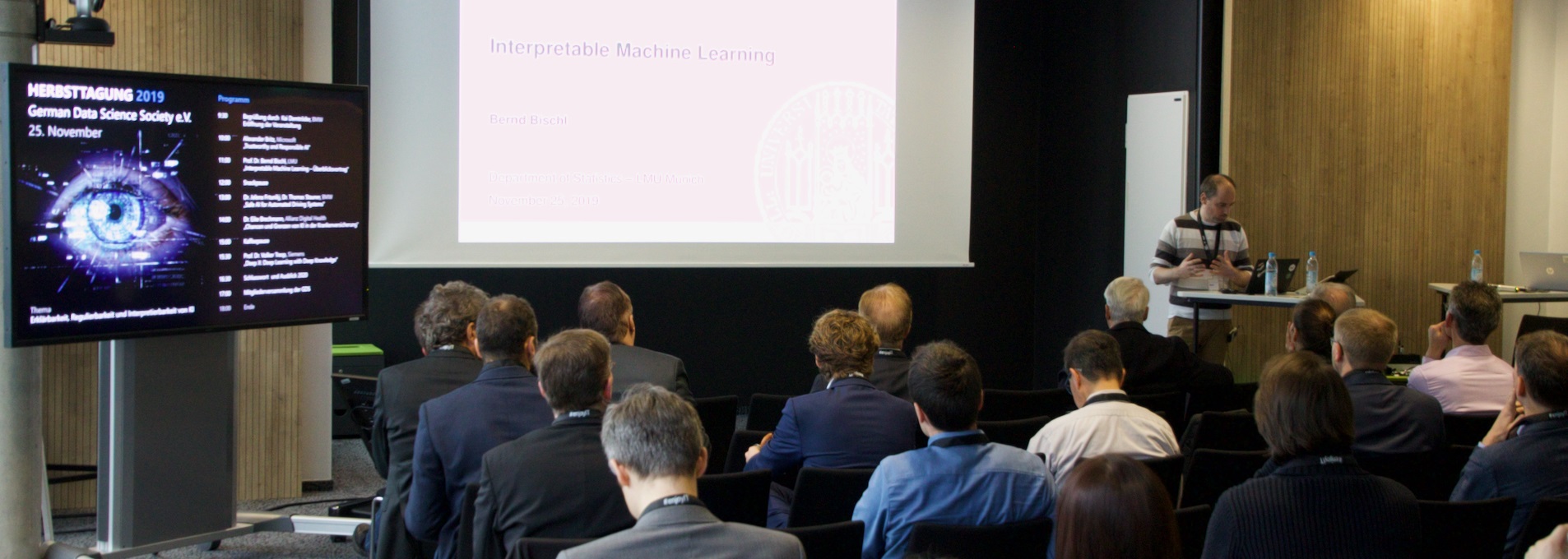
-
Bernd Bischl gave four main reasons for why it is important to explain AI:
Explain to justify
Helps argue for the use of AI in certain situations and use cases and increases trust in modelsExplain to control
Provides accountability, especially when a model can be used for consequential decisions about humans, for example lending or hiring decisionsExplain to improve
For debugging machine learning modelsExplain to discover
Understand how a model identifies patterns
Interpretability can mean one of two things:
Global interpretability means understanding the overall properties of a model: you can see which features of the model influence its predictions in which way.
Local interpretability, in contrast, means reviewing one specific outcome and understanding how the model arrived at this very prediction.He presented a few tools to interpret models, ranging from model-specific to model-agnostic methods.
Safe AI for Automated Driving by Jelena Frtunikj and Thomas Stauner (BMW)
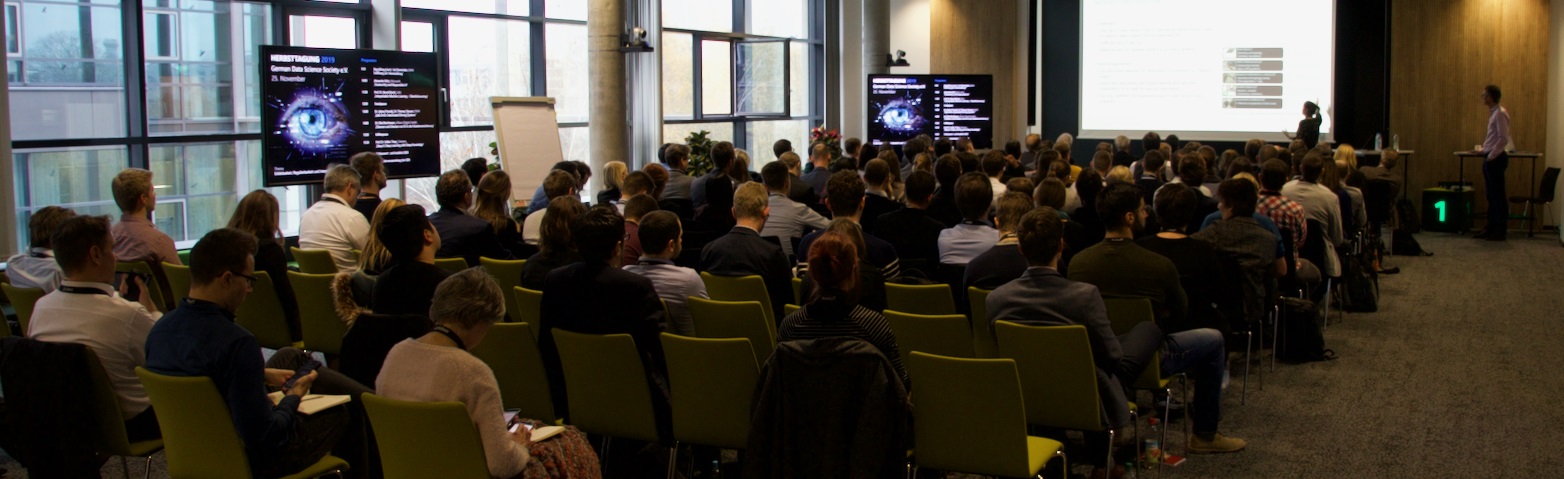
BMW focuses on supervised deep learning for object detection. They use a mix of real and synthetic images to train the model to recognize humans, animals, vehicles and traffic signs.
The model is only allowed 40 milliseconds to interpret the image and make a decision.
Developing AI for automated driving does not only require good average precision. It is equally important to detect critical unusual situations. This rests on having high-quality training data that capture myriad situations and are labeled correctly and comprehensively.
The model needs to be built in a way that it can react appropriately when one rule conflicts with another. Think, for example, about a pedestrian who crosses at red even though a car approaches. Here, the principle ‘protect humans’ conflicts with the rule ‘green light means go.’
Opportunities and Challenges of AI in Health Insurance by Eike Brechmann (Allianz Digital Health)
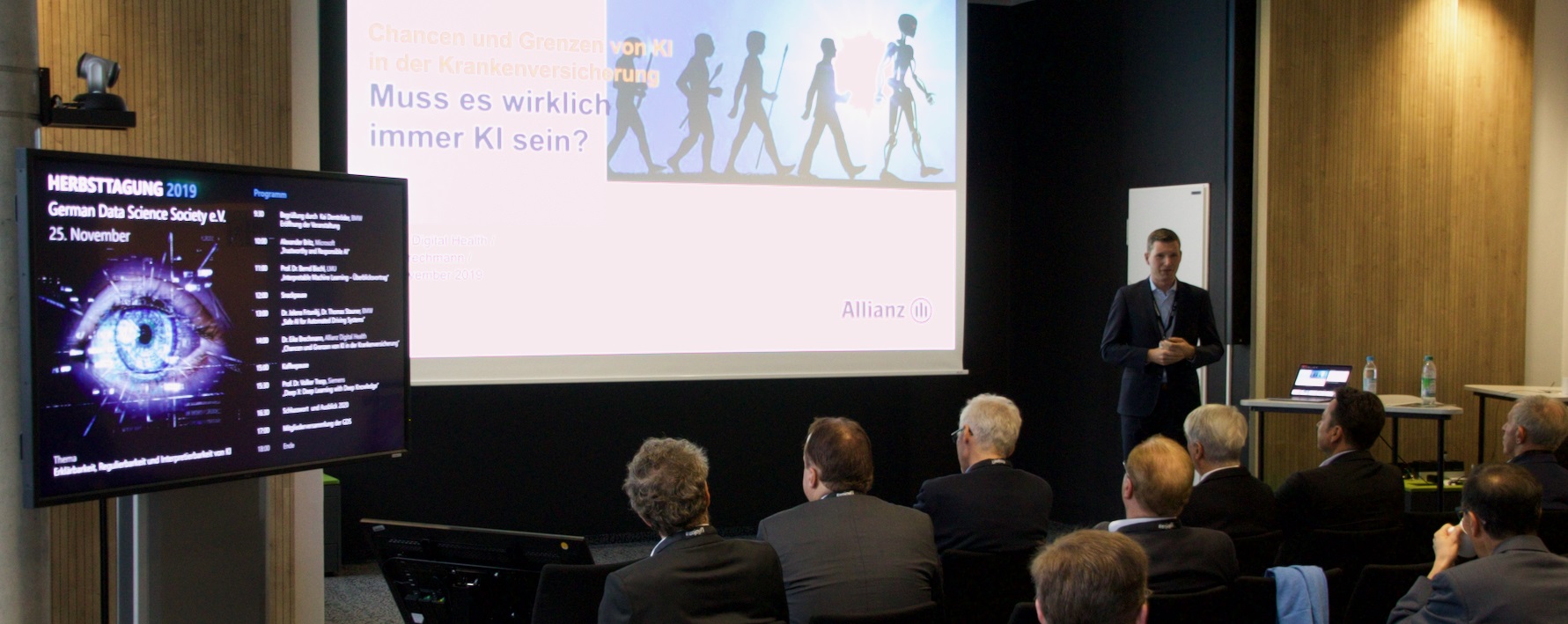
Insurance has always been a data-driven business model. AI has a huge potential to drive business impact when we improve our processes.
Eike presented four use cases for AI in health insurance. The central theme in all four was connecting a medical diagnosis to expenses on the part of the insurer. This can simplify processes and provide helpful inputs for claims handlers and health professionals – if the results are explainable and interpretable for Allianz employees, customers and regulators.
Use case 1:
Assessing and quantifying the cost risk of pre-existing conditions. Imagine a 25-year old who has hay fever wants to take out insurance with us. What is their risk of developing asthma, for example, and how will this reflect in the overall cost when we will likely insure them for more than 50 years?Use case 2:
How can we detect potential fraud, for example when a client does not declare their pre-existing conditions at the time the contract is signed?Use case 3:
How can we identify instances in which health care providers manipulate invoices and provide unjustifiably expensive treatments or services? In this case, unsupervised machine learning can detect deviations from what would be considered the usual treatment and expenses for a given illness.Use case 4:
AI can help identify customer groups who are at higher risk for certain illnesses and help them take preventive action.
DeepX: Deep Learning with Deep Knowledge by Volker Tresp (Siemens)
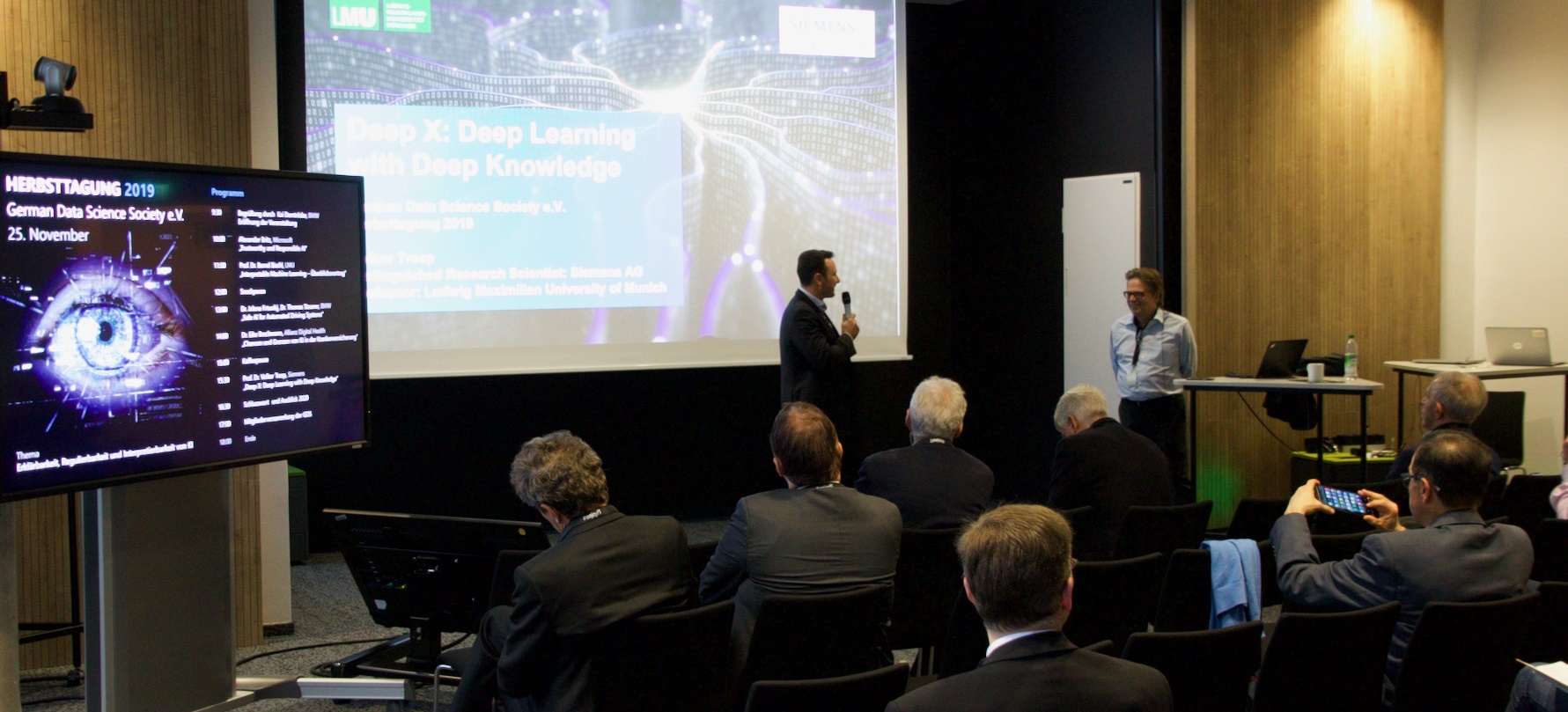
- Volker talked about his research in the area of combining knowledge graphs with deep learning. A knowledge graph is a graphical representation of information that shows relations between objects, people, events, etc.
- By combining the two, a model can not only identify objects in an image, but also their relationship, for example two humans side by side.
- Using knowledge graphs, a model can even recognize relationships that were not present in the training data. One example: Imagine we want to train a machine learning model to recognize humans, car and bicycles, and what their relation is in the image, e.g. human-on-bicycle. The model has to ‘learn’ first what humans in cars and on bicycles look like, and this relies on having a sufficient amount of training data. Our data may contain images of a human next to a car and of a human on a bicycle, but we are lacking images of humans next to a bicycle. In this case, a knowledge graph can help the model learn that humans can, in fact, be next to bicycles, and what this looks like – even though it’s nowhere in the training images.
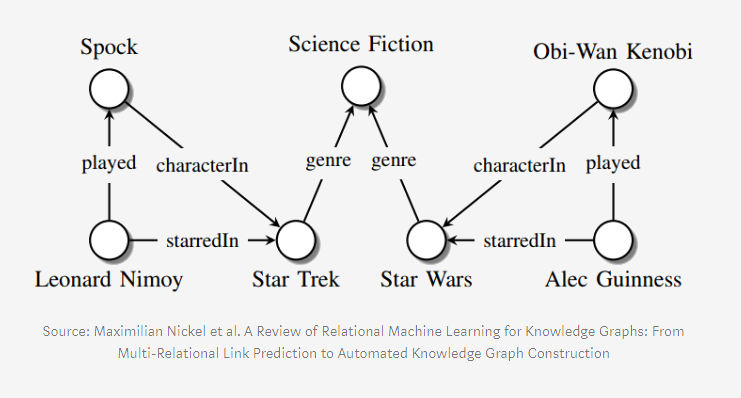